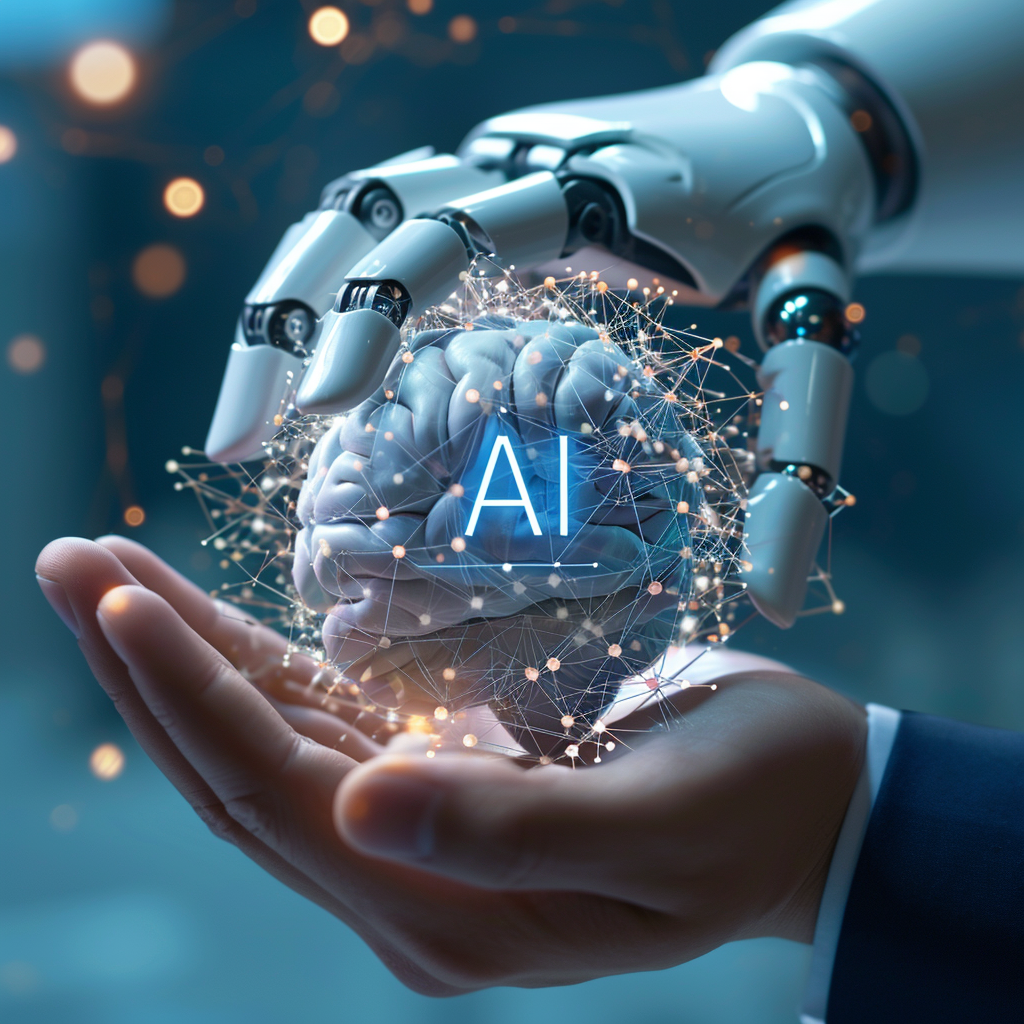
Picture this: You’re a marketing manager, excitedly implementing a new AI-powered lead generation system. You’ve heard amazing things about how it’ll transform your business. But a few months in, you’re scratching your head, wondering why you’re not seeing the results you expected. Sound familiar?
You’re not alone! AI lead generation is like a shiny new sports car – it looks fantastic and has incredible potential, but if you don’t know how to drive it properly, you might end up spinning your wheels.
Here’s a mind-boggling stat for you: 61% of marketers say generating traffic and leads is their top challenge. AI promises to be the superhero that saves the day, but like any good superhero story, there are villains to overcome. In our case, these villains are the challenges that come with implementing AI in lead generation.
But don’t worry! We’re about to embark on a journey through the AI lead generation landscape, identifying the obstacles and equipping you with the tools to overcome them. By the end of this article, you’ll be ready to navigate the AI lead gen terrain like a pro, leaving your competition in the dust.
Key Takeaways:
• Understand the critical AI lead generation challenges facing businesses in 2024
• Learn practical strategies to overcome data quality and integration issues
• Discover how to balance personalization with privacy concerns
• Gain insights on maintaining the human touch in AI-driven interactions
• Explore ethical considerations in AI lead generation and how to address them
Now, let’s dive into the nitty-gritty of AI lead generation challenges and how to conquer them!
Data Quality and Integration Issues: The Foundation of AI Success
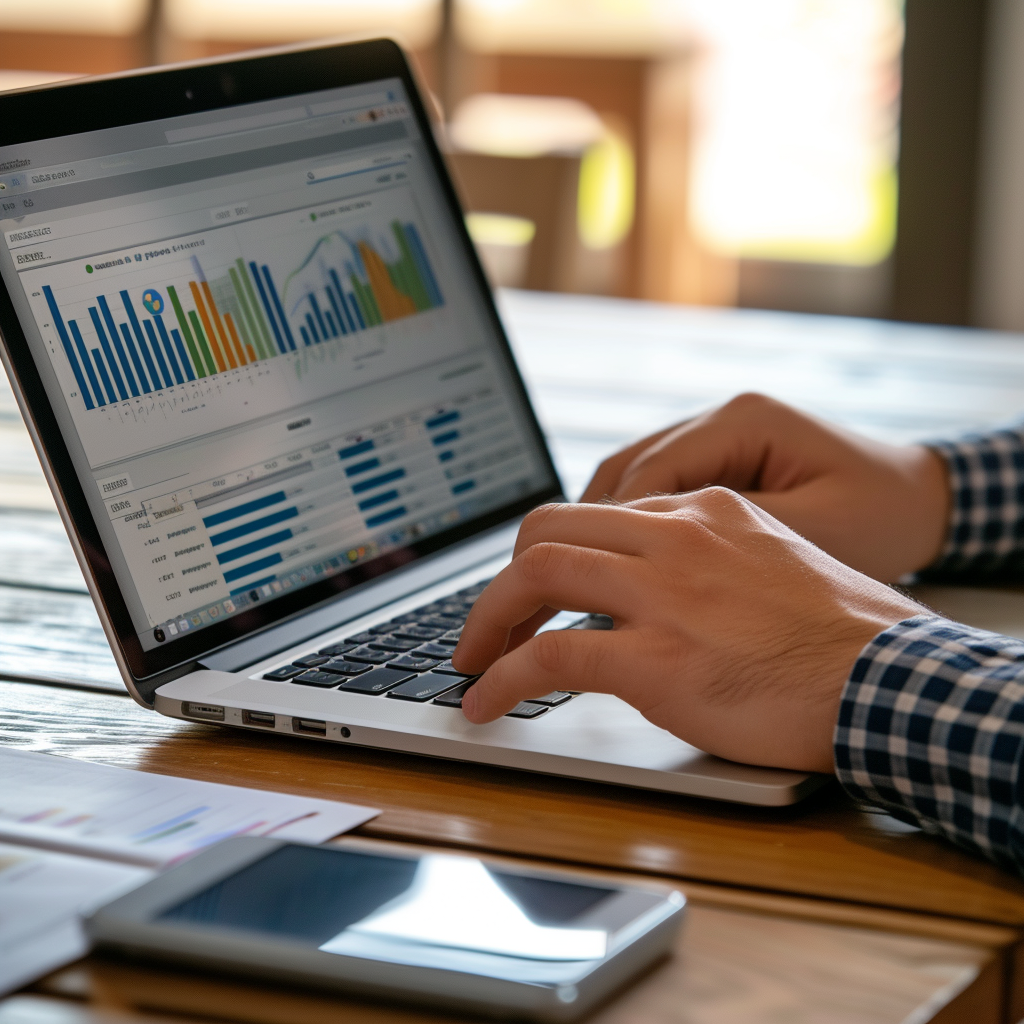
Imagine trying to build a sandcastle with mud instead of sand. That’s what using poor-quality data in AI lead generation is like. Your AI algorithms are only as good as the data you feed them.
The Challenge:
• Integrating data from multiple sources
• Ensuring data accuracy and completeness
• Maintaining consistent data formats across systems
How to Overcome It:
1. Implement rigorous data cleansing processes
2. Use data integration tools to unify information from various sources
3. Regularly audit your data for accuracy and completeness
Example: Let’s say you’re merging customer data from your CRM, email marketing platform, and social media analytics. Create a standardized format for names, addresses, and other key fields. Then, use a tool like Talend or Informatica to integrate and clean your data automatically.
As you clean up your data, you’ll start to see patterns emerge – patterns that will lead us to our next challenge: personalization.
Balancing Personalization and Privacy Concerns: Walking the Tightrope
Personalization in lead generation is like seasoning in cooking – the right amount can make your dish irresistible, but too much can ruin the whole meal.
The Challenge:
• Delivering personalized experiences without being creepy
• Complying with data protection regulations (GDPR, CCPA, etc.)
• Building trust with potential leads
How to Overcome It:
1. Be transparent about data collection and usage
2. Implement strong data protection measures
3. Offer clear opt-in/opt-out choices for personalized marketing
Example: Create a preference center where leads can choose what types of personalized content they want to receive. This not only respects their privacy but also improves the relevance of your marketing efforts.
As we perfect the art of personalization, we need to ensure we understand how our AI is making decisions. This brings us to our next challenge…
Overcoming the “Black Box” Problem in AI Decision-Making
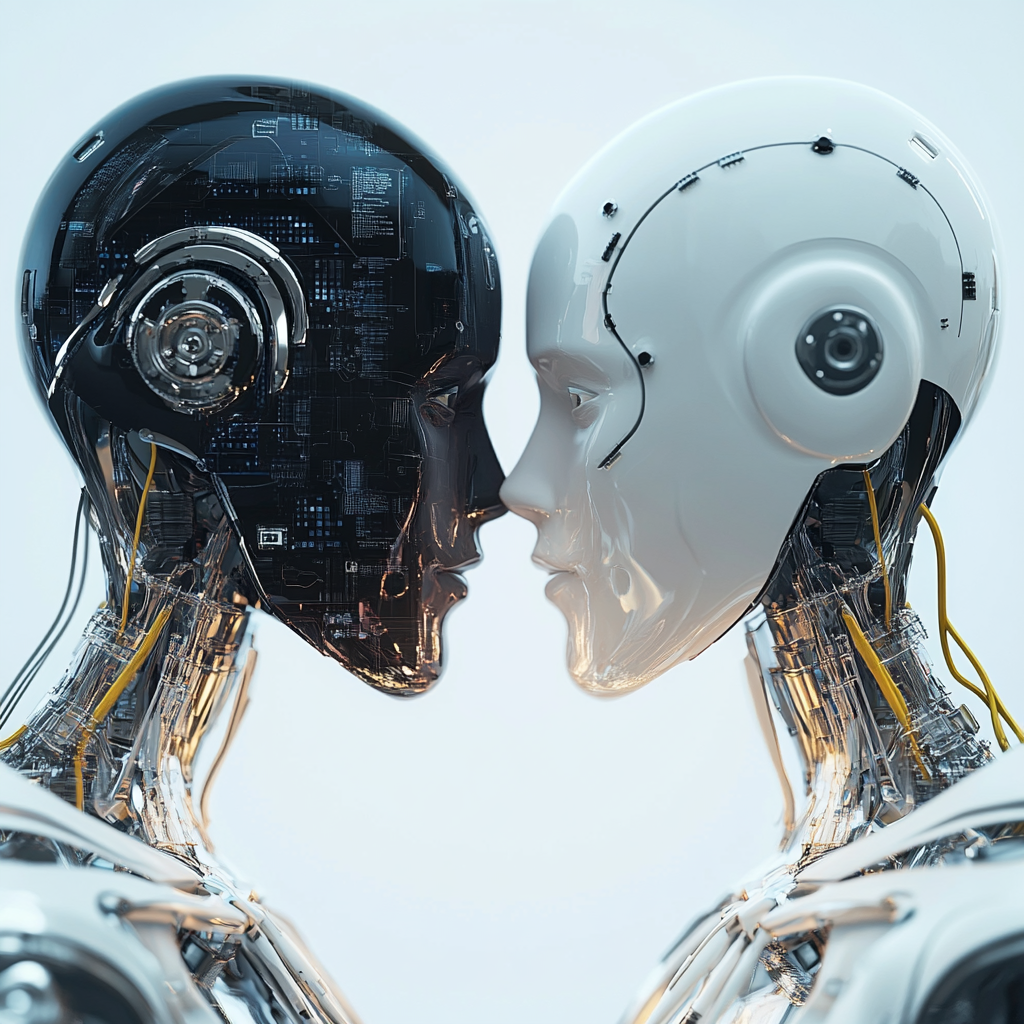
AI decision-making can sometimes feel like a magic trick – impressive, but mysterious. However, in the world of lead generation, we need to know how the rabbit got into the hat.
The Challenge:
• Understanding why AI makes certain lead scoring decisions
• Explaining AI-driven strategies to stakeholders
• Identifying and correcting errors in AI logic
How to Overcome It:
1. Use explainable AI tools that provide insights into decision-making processes
2. Regularly review and validate AI decisions against human expertise
3. Create clear documentation of AI models and their decision criteria
Example: Implement a tool like LIME (Local Interpretable Model-agnostic Explanations) to generate human-readable explanations for your AI’s lead scoring decisions. This allows you to verify the logic and make adjustments as needed.
As we shed light on the AI decision-making process, we uncover another critical challenge: bias.
Addressing Bias and Fairness in AI Lead Generation
AI bias is like a funhouse mirror – it can distort reality and lead us to make unfair decisions without us even realizing it.
The Challenge:
• Identifying and mitigating algorithmic bias in lead targeting
• Ensuring diverse representation in training data
• Avoiding discrimination in AI-driven lead generation
How to Overcome It:
1. Regularly audit your AI models for bias
2. Diversify your training data to include a wide range of demographics
3. Implement fairness constraints in your AI algorithms
Example: Use a tool like IBM’s AI Fairness 360 to check your lead scoring model for bias against protected characteristics like age, gender, or race. If bias is detected, adjust your model and training data accordingly.
Now that we’ve tackled bias, let’s look at the challenge of scaling our AI lead generation efforts.
Scaling AI Lead Generation Efforts: From Pilot to Powerhouse
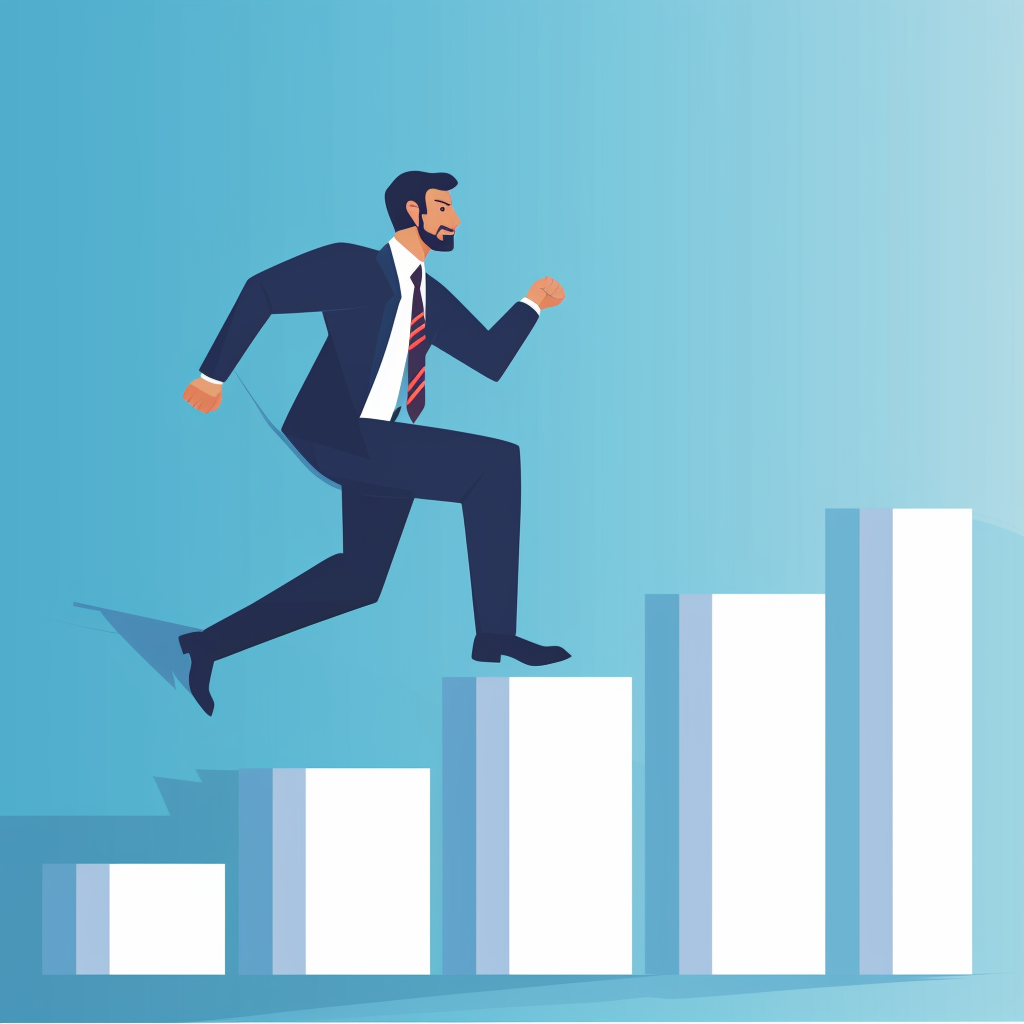
Scaling AI lead generation is like going from running a lemonade stand to managing a nationwide beverage company. The principles might be similar, but the execution is a whole different ball game.
The Challenge:
• Moving from successful pilot projects to full-scale implementation
• Allocating resources effectively for AI initiatives
• Demonstrating ROI to justify increased investment
How to Overcome It:
1. Develop a phased scaling plan with clear milestones
2. Invest in scalable infrastructure and tools
3. Continuously measure and communicate the impact of AI on lead generation metrics
Example: Start by fully automating one aspect of your lead generation process, such as lead scoring. Once that’s running smoothly, expand to automated lead nurturing, then to predictive lead identification. Track key metrics at each stage to demonstrate value.
As we scale up our AI efforts, we need to ensure our team can keep up. This leads us to our next challenge…
Bridging the Skills Gap in AI Marketing
The AI skills gap is like trying to sail a high-tech yacht with a crew trained for rowboats. We need to level up our team’s skills to match our AI ambitions.
The Challenge:
• Finding and retaining AI-savvy marketing professionals
• Upskilling existing team members in AI technologies
• Fostering collaboration between marketing and data science teams
How to Overcome It:
1. Invest in AI and machine learning training for your marketing team
2. Partner with universities or coding bootcamps for talent pipelines
3. Create cross-functional teams that blend marketing and data science expertise
Example: Organize an “AI for Marketers” bootcamp for your team. Bring in experts to teach the basics of machine learning, data analysis, and AI-driven marketing strategies. Follow up with hands-on projects to apply these new skills.
As our team becomes more AI-savvy, we need to ensure we don’t lose sight of the human element in lead generation.
Maintaining Human Touch in AI-Driven Interactions
Balancing AI automation with human interaction is like being a DJ – you need to know when to let the tech do its thing and when to step in and add that personal touch.
The Challenge:
• Avoiding robotic, impersonal interactions with leads
• Identifying when human intervention is necessary
• Creating AI-driven communications that feel authentic
How to Overcome It:
1. Use AI to augment human interactions, not replace them
2. Implement sentiment analysis to detect when leads need human attention
3. Craft AI-generated content that mirrors your brand’s voice and personality
Example: Create an AI chatbot for initial lead engagement, but program it to seamlessly hand off to a human sales rep when it detects complex questions or strong buying signals.
Now that we’ve mastered the art of human-AI collaboration, let’s tackle the challenge of measuring our success.
Measuring and Optimizing AI Lead Generation Performance
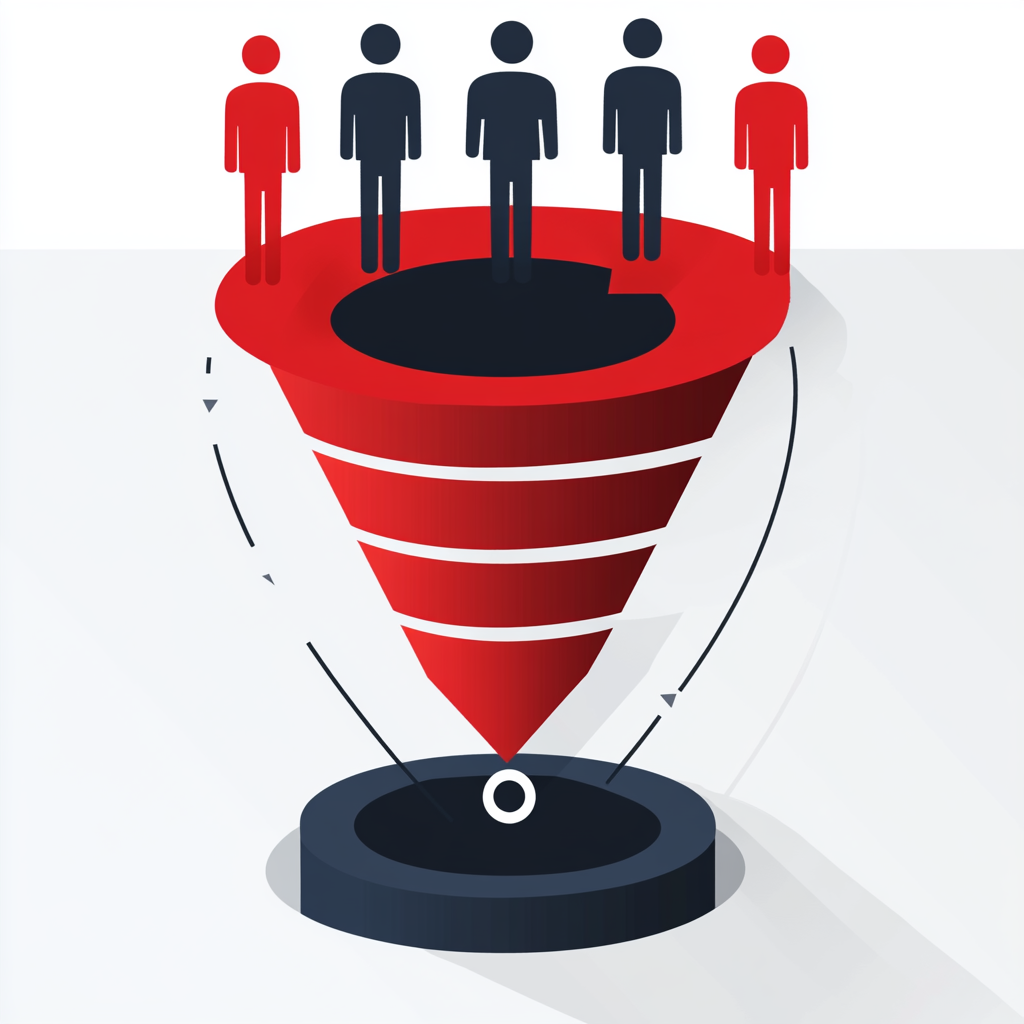
Measuring AI lead generation performance is like trying to weigh air – it’s essential, but not always straightforward.
The Challenge:
• Defining relevant KPIs for AI-driven lead gen initiatives
• Attributing success accurately between AI and traditional methods
• Continuously improving AI models based on performance data
How to Overcome It:
1. Establish clear, AI-specific KPIs (e.g., model accuracy, lead quality score)
2. Implement advanced attribution models that account for AI touchpoints
3. Use A/B testing to compare AI-driven strategies against traditional methods
Example: Set up a dashboard that tracks both traditional metrics (conversion rates, cost per lead) and AI-specific metrics (algorithm confidence scores, predictive accuracy). Use this to identify areas where AI is outperforming human efforts and vice versa.
As we optimize our AI performance, we need to keep an eye on the rapidly changing technology landscape.
Navigating the Rapidly Evolving AI Technology Landscape
Keeping up with AI advancements is like trying to drink from a firehose – there’s a lot coming at you, and it’s coming fast.
The Challenge:
• Staying informed about new AI tools and platforms
• Evaluating which new technologies are worth adopting
• Futureproofing your AI lead generation strategy
How to Overcome It:
1. Allocate time and resources for ongoing AI education and exploration
2. Develop partnerships with AI vendors and research institutions
3. Create an agile AI adoption framework that allows for quick pivots
Example: Establish a quarterly “AI Innovation Day” where your team explores new AI technologies and brainstorms potential applications for your lead generation efforts.
As we embrace new AI technologies, we must also grapple with the ethical implications of our choices.
Conclusion:
Whew! We’ve just taken a whirlwind tour through the top 10 AI lead generation challenges of 2024. It’s been quite a ride, hasn’t it? From wrangling data to wrestling with ethical dilemmas, the path to AI-powered lead generation success is filled with twists and turns.
But here’s the exciting part: each challenge we’ve discussed is also an opportunity. An opportunity to refine your processes, to understand your leads better, and to create more meaningful connections with your potential customers.
Remember, AI in lead generation isn’t about replacing the human touch – it’s about enhancing it. It’s about using technology to free up your team to do what humans do best: build relationships, solve complex problems, and drive innovation.